CUDA Driver and Runtime Compatibility
Hierarchical Architecture & Core Concepts
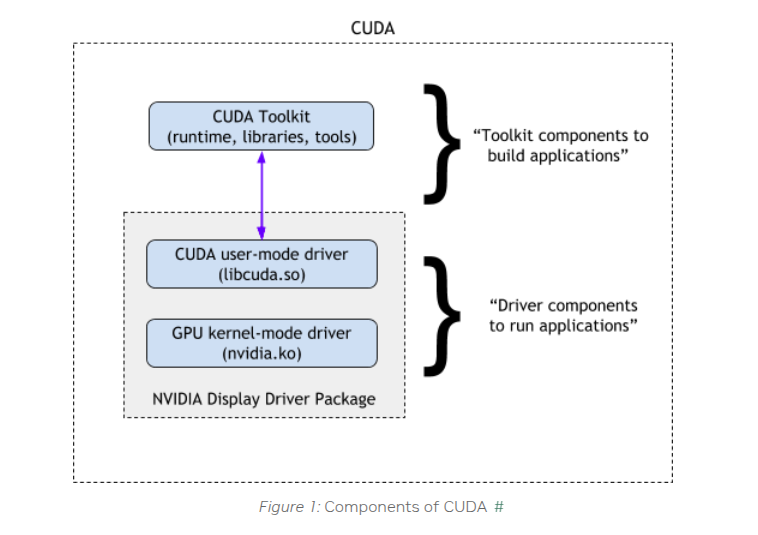
1. CUDA Runtime API Layer
Technical Positioning
- Functional Scope: Provides high-level abstraction interfaces for developers, encapsulating core GPU operations (memory allocation, stream management, kernel launches, etc.)
- Version Binding: Determined by the CUDA Toolkit version used during build (e.g., CUDA 12.0.1)
Version Detection Methods
# Python environment detection (recommended priority method)
pip list | grep cuda
conda list |grep cuda
# Example output: cu121 # cu121 indicates CUDA 12.1 environment
# System-level runtime library detection
find / -name "libcudart*"
# cudart means cuda runtime
# Example output:
/usr/local/cuda-12.4/targets/x86_64-linux/lib/libcudart.so.12
/usr/local/cuda-12.4/targets/x86_64-linux/lib/libcudart.so.12.4.127
Indicates CUDA 12.4
if you find multi lib version, you should check your program which version used,like PATH, LD_LIBRARY_PATH or other program set
env |grep PATH
# Example output:
LIBRARY_PATH=/usr/local/cuda/lib64/stubs
LD_LIBRARY_PATH=/usr/local/nvidia/lib:/usr/local/nvidia/lib64
PATH=/go/bin:/usr/local/go/bin:/usr/local/nvidia/bin:/usr/local/cuda/bin:/usr/local/sbin:/usr/local/bin:/usr/sbin:/usr/bin:/sbin:/bin
#that means your cuda program used the first lib by lib path order
2. CUDA Driver API Layer
Technical Positioning
- Functional Scope: Low-level interface directly interacting with GPU hardware, handling instruction translation and hardware resource scheduling
- Version Binding: Determined by NVIDIA driver version, following SemVer specification
Version Detection Methods
nvidia-smi
# Example output:
+-----------------------------------------------------------------------------------------+
| NVIDIA-SMI 550.144.03 Driver Version: 550.144.03 CUDA Version: 12.4 |
|-----------------------------------------+------------------------+----------------------+
| GPU Name Persistence-M | Bus-Id Disp.A | Volatile Uncorr. ECC |
| Fan Temp Perf Pwr:Usage/Cap | Memory-Usage | GPU-Util Compute M. |
| | | MIG M. |
|=========================================+========================+======================|
| 0 NVIDIA A30 Off | 00000000:00:0B.0 Off | 0 |
| N/A 31C P0 28W / 165W | 10195MiB / 24576MiB | 0% Default |
| | | Disabled |
+-----------------------------------------+------------------------+----------------------+
Version Compatibility Matrix & Constraints
Physical GPU Deployment - Core Compatibility Principles
First reference NVIDIA's official statement,the base constraints is
- Driver version must always be ≥ Runtime version
- NVIDIA officially guarantees 1 major version backward compatibility (e.g., CUDA Driver 12.x supports Runtime 11.x)
- Cross-two-major-version compatibility (e.g., Driver 12.x with Runtime 10.x) is neither officially supported nor recommended
when you deploy cuda program,please comply with the base constraints
Formal Rules
+ Mandatory: Driver version ≥ Runtime version
+ Recommended: Driver major version - Runtime major version ≤ 1
- Blocked: Driver version < Runtime version → May trigger CUDA_ERROR_UNKNOWN(999)
- Unstable: Driver major version - Runtime major version > 1 → Application may malfunction
Virtualization Scenario Enhancements (HAMI/GPU-Manager)
When using Virtual GPU solutions like GPU-Manager or HAMI, besides the base constraints up, you must comply with the additional constraints apply:
Version Requirements
1. Virtual GPU solutions baseline version ≥ Runtime version
2. Runtime major version = Driver major version = Baseline major version
Special Note for GPU-Manager:
We implemented partial cross-1-major-version compatibility (e.g., baseline 12.4 supporting vLLM 11.8). However, this requires per-application hook adjustments and must be analyzed case-by-case.
Deployment Best Practices
Recommended Strategy
• Adopt newer CUDA versions (e.g., CUDA 12.x) for both Driver and Runtime in new GPU cluster planning
Alternative Solutions for Legacy Systems
1. Physical GPU Scheduling or GPU-Manager Whole-Card Allocation
Whole-card scheduling provides native compatibility equivalent to physical GPU access
GPU-Manager can use whole card mode when you set tencent.com/vcuda-core to 100 multily positive integer,like 100,200,300
resources:
limits:
tencent.com/vcuda-core: "100"
2. Node Labeling Strategy
Label nodes based on supported Driver CUDA versions:
node_labels:
cuda-major-version: "12"
cuda-minor-version: "4"
this means your node is cuda 12.4
Configure scheduling affinity in deployments:
you can set cuda-major-version and cuda-minor-version by your program cuda runtime need
apiVersion: apps/v1
kind: Deployment
metadata:
name: cuda-app
spec:
template:
spec:
affinity:
nodeAffinity:
requiredDuringSchedulingIgnoredDuringExecution:
nodeSelectorTerms:
- matchExpressions:
- key: cuda-major-version
operator: In
values: ["12"]
- key: cuda-minor-version
operator: Gt
values: ["2"]
3. Runtime Version Upgrade
Legacy CUDA Runtimes may have security vulnerabilities (CVEs) and lack support for new GPU features. Prioritize upgrades to CUDA 12.x.
nvidia recomend to upgrade both
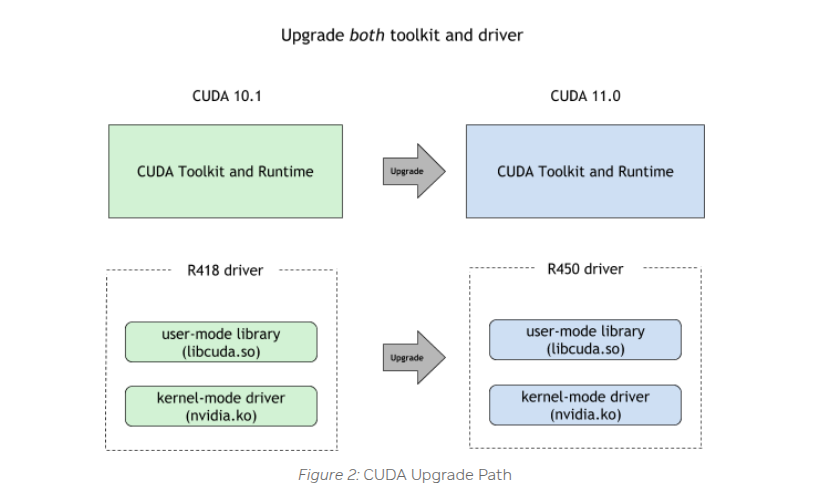
Troubleshooting Handbook
Common Error Codes
Error Code | Description | Recommended Action |
---|
CUDA_ERROR_INVALID_IMAGE | Driver-Runtime incompatibility | Align driver version with container CUDA version |
CUDA_ERROR_ILLEGAL_ADDRESS | Virtual memory violation (common in version mismatch) | Verify Runtime vs baseline constraints |
CUDA_ERROR_UNSUPPORTED_PTX_VERSION | PTX instruction set mismatch | Recompile with explicit -arch=sm_xx |